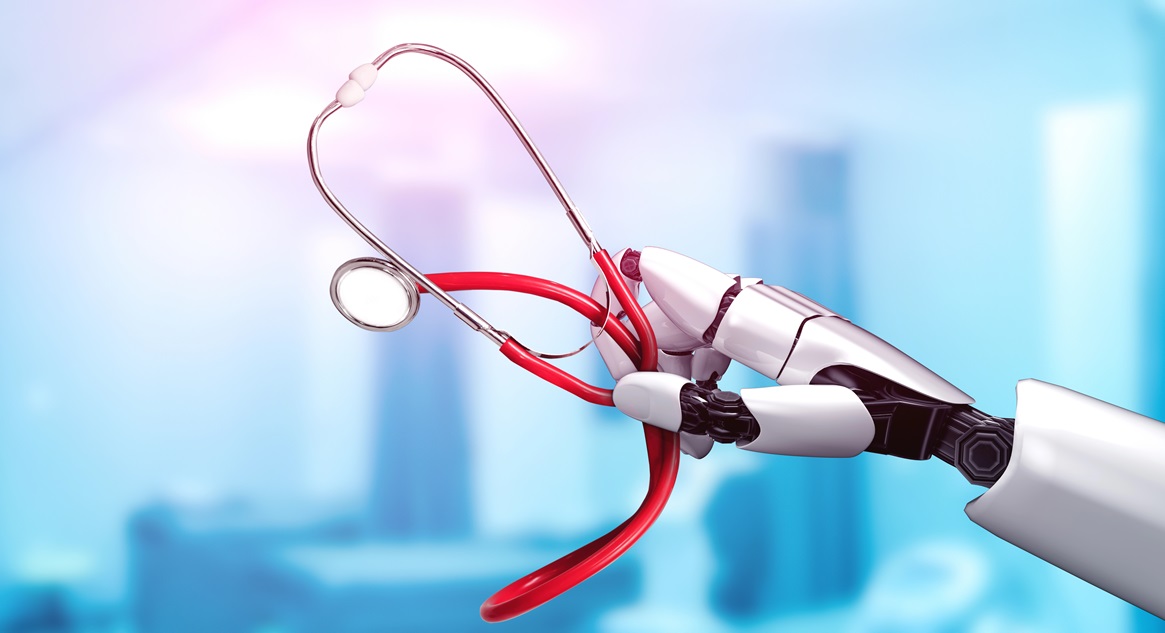
The AI Revolution in Healthcare: Investing in the Future of Generative AI-Powered Medical Solutions
Introduction
The rise of generative AI in healthcare
The integration of generative AI in healthcare has been rapidly accelerating, driven by advancements in machine learning algorithms and the availability of vast medical datasets. This technological revolution promises to transform various aspects of medical practice, including diagnosis, treatment planning, and personalized patient care.
Overview of the current landscape
The current landscape of generative AI in healthcare is characterized by rapid advancements in machine learning algorithms and the increasing availability of large-scale medical datasets. These developments have enabled AI systems to analyze complex medical information, generate insights, and effectively support clinical decision-making. However, integrating generative AI into routine clinical practice faces significant challenges, including ethical considerations, technical limitations, and the need for robust validation in real-world medical settings.
Understanding Generative AI in Healthcare
Definition and key concepts
Types of generative AI models used in healthcare
Natural Language Processing (NLP) models
Natural Language Processing (NLP) models, such as large language models (LLMs), have emerged as powerful tools in healthcare. These models can analyze and generate human-like text based on vast amounts of medical data. These models can facilitate seamless healthcare conversations, supporting applications ranging from patient diagnosis to treatment suggestions and healthcare provider support systems.
Image generation and analysis models
Image generation and analysis models in healthcare leverage deep learning techniques to create and interpret medical images with unprecedented accuracy. These models, such as Generative Adversarial Networks (GANs), have demonstrated remarkable capabilities in synthesizing realistic medical images, enhancing image quality, and aiding in tasks like image segmentation and disease detection. By generating synthetic medical data, these models address the critical challenge of limited datasets in healthcare, facilitating improved diagnostics, research, and training for medical professionals.
Predictive and diagnostic models
Predictive and diagnostic models in healthcare leverage machine learning algorithms to analyze vast amounts of patient data, identifying patterns and risk factors that may indicate the onset or progression of diseases. These models have demonstrated remarkable accuracy in early disease detection and prognosis, particularly in complex conditions such as sepsis, where timely intervention is crucial. By integrating diverse data sources, including electronic health records, genetic information, and real-time patient monitoring data, these AI-driven systems enable healthcare providers to make more informed decisions and implement personalized treatment strategies.
Applications of Generative AI in Healthcare
Clinical decision support systems
Clinical decision support systems (CDSS) powered by generative AI are revolutionizing medical practice by providing real-time, data-driven insights to healthcare professionals. These systems analyze vast patient data, including electronic health records, genetic information, and medical imaging, to generate personalized treatment recommendations and risk assessments. However, while CDSS shows promise in enhancing clinical decision-making, their integration into routine practice requires careful oversight and validation to ensure patient safety and optimal outcomes.
Medical imaging and diagnostics
In the realm of medical imaging and diagnostics, generative AI models have demonstrated remarkable capabilities in enhancing image quality, detecting anomalies, and assisting radiologists in their interpretations. These AI-powered systems can analyze complex medical images, such as MRIs, CT scans, and X-rays, with unprecedented accuracy and speed, potentially leading to earlier detection of diseases and improved patient outcomes. Furthermore, the integration of AI in medical imaging has paved the way for innovative applications like digital twins, which create virtual replicas of physical systems, enabling predictive maintenance and accelerating the training of machine learning models in healthcare settings.
Drug discovery and development
Generative AI has emerged as a powerful tool in drug discovery and development, offering the potential to significantly accelerate the process and reduce costs. By leveraging machine learning algorithms and vast chemical databases, these AI models can predict molecular structures, optimize lead compounds, and simulate drug-target interactions with unprecedented speed and accuracy. This technology has already demonstrated promising results in identifying novel drug candidates for various diseases, potentially revolutionizing the pharmaceutical industry’s approach to drug development to accelerate the process and reduce costs significantly
Personalized treatment plans
Generative AI models are revolutionizing personalized treatment plans by analyzing vast patient data to tailor interventions to individual needs and genetic profiles. These AI-driven systems can integrate diverse data sources, including electronic health records, genetic information, and real-time patient monitoring data, to generate highly specific treatment recommendations for complex interactions between patient characteristics and potential therapies.
Virtual health assistants and chatbots
These AI-powered virtual health assistants leverage natural language processing and machine learning algorithms to provide personalized healthcare support, ranging from symptom assessment to medication reminders. By offering 24/7 accessibility and reducing the burden on healthcare providers, virtual assistants can potentially improve patient engagement and streamline healthcare delivery, particularly in remote or underserved areas.
Market Analysis of AI-Focused Healthcare Companies
Current market size and growth projections
The global AI in healthcare market is projected to reach $187.95 billion by 2030, with a compound annual growth rate (CAGR) of 37.5% from 2022 to 203. This substantial growth is driven by increasing investments in AI-powered medical solutions and the rising demand for personalized healthcare services.
Key players and emerging startups
Among the key players in the AI-powered healthcare market, companies like IBM Watson Health, Google Health, and Microsoft Healthcare have established themselves as frontrunners. Emerging startups such as Tempus, Freenome, and Recursion Pharmaceuticals are also making significant strides in developing innovative AI solutions for personalized medicine, early disease detection, and drug discovery.
Investment trends and funding patterns
Investment trends in AI-powered healthcare solutions have shown a significant uptick, with venture capital funding reaching $8.5 billion in 2022, representing a 35% increase from the previous year. This surge in funding is particularly notable in areas such as generative AI for drug discovery, AI-assisted diagnostic imaging, and personalized treatment planning.
Conclusion
The transformative potential of generative AI in healthcare
The surge in funding for AI-powered healthcare solutions is particularly evident in the realm of digital twins, which are being leveraged to create virtual replicas of physical products, processes, or systems in healthcare settings. These digital twins are not only enhancing predictive maintenance in medical equipment but also accelerating the training of machine learning AI systems by generating vast, quality datasets for healthcare applications.
Balancing risks and rewards in long-term investments
The surge in funding for AI-powered healthcare solutions is particularly notable in the field of drug discovery, where generative AI models are being employed to accelerate the identification of novel compounds and optimize molecular structures. This application of generative AI has the potential to significantly reduce the time and cost associated with traditional drug development processes, potentially leading to faster and more efficient creation of life-saving medications.
References
Chow, J. C. L., Wong, V., & Li, K. (2024). Generative Pre-Trained Transformer-Empowered Healthcare Conversations: Current Trends, Challenges, and Future Directions in Large Language Model-Enabled Medical Chatbots.
Satyanarayan Kanungo, Tolu Adedoja, Sourabh Sharma, S. D., Suman Narne, Sathishkumar Chintala,. (2024). Exploring AI-driven Innovations in Image Communication Systems for Enhanced Medical Imaging Applications.
Sriram Sitaraman. (2024). AI-Driven Diagnostics and Imaging: Transforming Early Detection and Precision in Healthcare.
Sai, S., Gaur, A., Sai, R., Chamola, V., Guizani, M., & Rodrigues, J. J. P. C. (2024). Generative AI for Transformative Healthcare: A Comprehensive Study of Emerging Models, Applications, Case Studies, and Limitations.